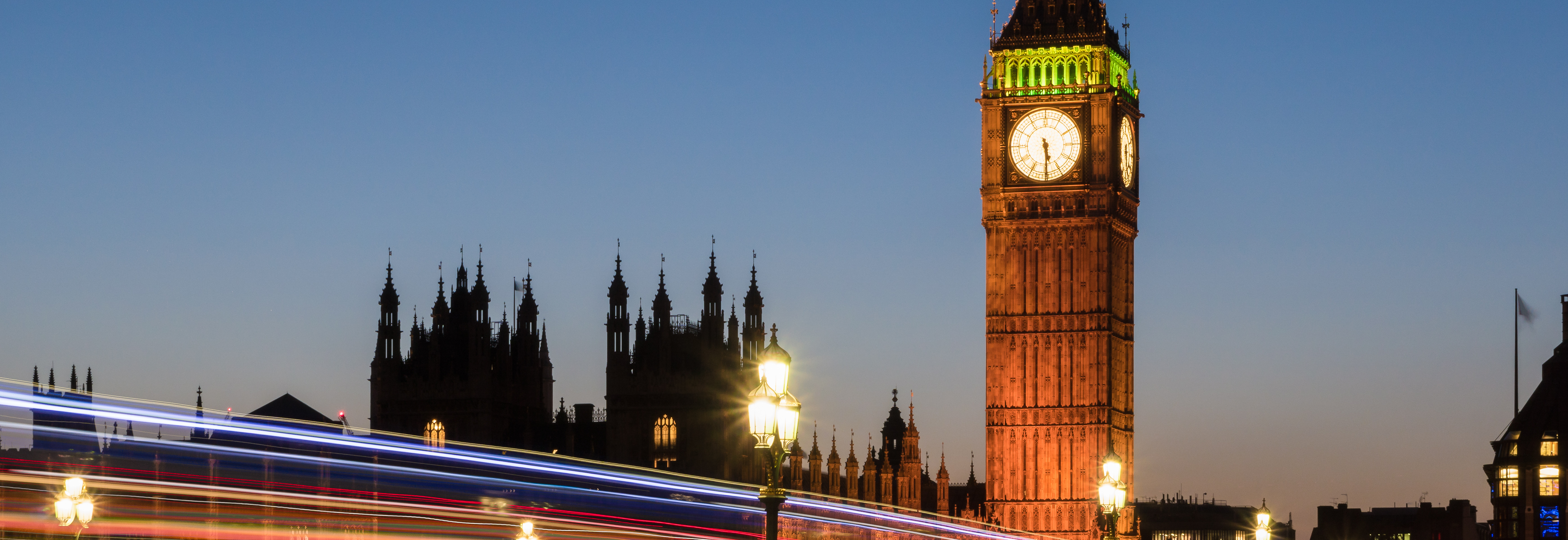
ALA 2023
29 & 30 May 2023, London, UK
News
- 24 June: Best paper is announced in the awards.
- 8 May 2023: Camera-ready copies of all papers are viewable in the program.
- 4 May 2023: Stay tuned for the camera-ready PDF's of each paper, which will be available on the website shortly.
- 3 May 2023: The program for the workshop is now available.
- 3 May 2023: We are excited to announce Shimon Whiteson as a keynote speaker for ALA 2023.
- 25 April 2023: We are excited to announce Peter Stone as a keynote speaker for ALA 2023.
- 21 April 2023: We are excited to announce Christopher Amato as a keynote speaker for ALA 2023.
- 17 April 2023: Information regarding the Neural Computing & Applications Journal Special Issue and Best Paper Award are now posted.
- 31 March 2023: The list of accepted papers is now online.
- 24 March 2023: Stay tuned! ALA 2023 acceptances will be announced on March 27th.
- 24 Jan 2023: ALA 2023 submission deadline has been extended to 24 Feb 2023 23:59 UTC
- 22 Dec 2022: ALA 2023 Submission Link can be found here
- 22 Dec 2022: ALA Call for Papers can be found here
- 22 Dec 2022: ALA 2023 Website goes live!
ALA 2023 - Workshop at AAMAS 2023
Adaptive and Learning Agents (ALA) encompasses diverse fields such as Computer Science, Software Engineering, Biology, as well as Cognitive and Social Sciences. The ALA workshop will focus on agents and multiagent systems which employ learning or adaptation.
This workshop is a continuation of the long running AAMAS series of workshops on adaptive agents, now in its fifteenth year. Previous editions of this workshop may be found at the following urls:
- ALA-22
- ALA-21
- ALA-20
- ALA-19
- ALA-18
- ALA-17
- ALA-16
- ALA-15
- ALA-14
- ALA-13
- ALA-12
- ALA-11
- ALA-10
- ALA-09
- ALAMAS+ALAg-08
- ALAg-07
- Earlier editions
The goal of this workshop is to increase awareness of and interest in adaptive agent research, encourage collaboration and give a representative overview of current research in the area of adaptive and learning agents and multi-agent systems. It aims at bringing together not only scientists from different areas of computer science (e.g. agent architectures, reinforcement learning, evolutionary algorithms) but also from different fields studying similar concepts (e.g. game theory, bio-inspired control, mechanism design).
The workshop will serve as an inclusive forum for the discussion of ongoing or completed work covering both theoretical and practical aspects of adaptive and learning agents and multi-agent systems.
This workshop will focus on all aspects of adaptive and learning agents and multi-agent systems with a particular amphasis on how to modify established learning techniques and/or create new learning paradigms to address the many challenges presented by complex real-world problems. The topics of interest include but are not limited to:
- Novel combinations of reinforcement and supervised learning approaches
- Integrated learning approaches that work with other agent reasoning modules like negotiation, trust models, coordination, etc.
- Supervised multi-agent learning
- Reinforcement learning (single- and multi-agent)
- Novel deep learning approaches for adaptive single- and multi-agent systems
- Multi-objective optimisation in single- and multi-agent systems
- Planning (single- and multi-agent)
- Reasoning (single- and multi-agent)
- Distributed learning
- Adaptation and learning in dynamic environments
- Evolution of agents in complex environments
- Co-evolution of agents in a multi-agent setting
- Cooperative exploration and learning to cooperate and collaborate
- Learning trust and reputation
- Communication restrictions and their impact on multi-agent coordination
- Design of reward structure and fitness measures for coordination
- Scaling learning techniques to large systems of learning and adaptive agents
- Emergent behaviour in adaptive multi-agent systems
- Game theoretical analysis of adaptive multi-agent systems
- Neuro-control in multi-agent systems
- Bio-inspired multi-agent systems
- Applications of adaptive and learning agents and multi-agent systems to real world complex systems
Extended and revised versions of papers presented at the workshop will be eligible for inclusion in a journal special issue (see below).
Important Dates
Submission Details
Papers can be submitted through EasyChair.
We invite submission of original work, up to 8 pages in length (excluding references) in the ACM proceedings format (i.e. following the AAMAS formatting instructions). This includes work that has been accepted as a poster/extended abstract at AAMAS 2023. Keeping with previous ALA guidelines, papers are limited to 8 pages plus references - no supplementary material is accepted. Additionally, we welcome submission of preliminary results, i.e. work-in-progress, as well as visionary outlook papers that lay out directions for future research in a specific area, both up to 6 pages in length, although shorter papers are very much welcome, and will not be judged differently. Finally, we also accept recently published journal papers in the form of a 2 page abstract.
Furthermore, for submissions that were rejected or accepted as extended abstracts at AAMAS, we encourage authors to also append the received reviews. This is simply a recommendation and it is optional. Authors can also include a short note or changelist they carried out on the paper. The reviews can be appended at the end of the submission file and do not count towards the page limit.
All submissions will be peer-reviewed (single-blind). Accepted work will be allocated time for poster and possibly oral presentation during the workshop. Extended versions of original papers presented at the workshop will also be eligible for inclusion in a post-proceedings journal special issue.
When preparing your submission for ALA 2023, please be sure to remove the AAMAS copyright block, citation information and running headers. Please replace the AAMAS copyright block in the main.tex file from the AAMAS template with the following:
\setcopyright{none} \acmConference[ALA '23]{Proc.\@ of the Adaptive and Learning Agents Workshop (ALA 2023)} {May 9-10, 2023}{Online, \url{https://ala2023.github.io/}}{Cruz, Hayes, Wang, Yates (eds.)} \copyrightyear{2023} \acmYear{2023} \acmDOI{} \acmPrice{} \acmISBN{} \settopmatter{printacmref=false}
Journal Special Issue
We are delighted to announce that extended versions of all original contributions at ALA 2023 will be eligible for inclusion in a special issue of the Springer journal Neural Computing and Applications (Impact Factor 5.130). The deadline for submitting extended papers will be 15 November 2023.
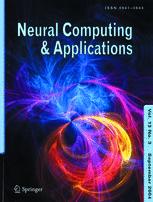
For further information please contact the workshop organizers and Patrick Mannion.
Program
All times are presented in local London time.
Monday May 29
Welcome & Opening Remarks | |
08:30-10:00 | Session I - Chair: Fernando P. Santos |
08:30-09:30 | Invited Talk: Shimon Whiteson (Oxford) Efficient & Realistic Simulation for Autonomous Driving |
09:30-09:45 | Long Talk: Raphael Avalos, Florent Delgrange, Ann Nowe, Guillermo A. Pérez and Diederik M. Roijers The Wasserstein Believer: Learning Belief Updates for Partially Observable Environments through Reliable Latent Space Models |
09:45-10:00 | Long Talk: Felipe Leno Da Silva, Jiachen Yang, Mikel Landajuela, Andre Goncalves, Alexander Ladd, Daniel Faissol and Brenden Petersen Toward Multi-Fidelity Reinforcement Learning for Symbolic Optimization |
10:00-11:00 | Coffee Break |
11:00-12:30 | Session II - Chair: Patrick Mannion |
11:00-11:15 | Long Talk: Minae Kwon, John Agapiou, Edgar Duéñez-Guzmán, Romuald Elie, Georgios Piliouras, Kalesha Bullard and Ian Gemp Aligning Local Multiagent Incentives with Global Objectives |
11:15-11:30 | Matthew E. Taylor Reinforcement Learning Requires Human-in-the-Loop Framing and Approaches |
11:30-12:30 | Short Talks, 6 minutes each in order:
|
12:30-14:00 | Lunch Break |
14:00-15:45 | Short Talks & Poster Session - Chair: Gaurav Dixit |
14:00-14:15 | Short Talks, 6 minutes each in order:
|
14:15-15:45 | Poster Session A |
15:45-16:30 | Coffee Break |
16:30-18:00 | Session III - Chair: Caroline Wang |
16:30-16:45 | Long Talk: Rory Lipkis and Adrian Agogino Discovery and Analysis of Rare High-Impact Failure Modes Using Adversarial RL-Informed Sampling |
16:45-17:00 | Long Talk: Montaser Mohammedalamen, Dustin Morrill, Alexander Sieusahai, Yash Satsangi and Michael Bowling Learning to Be Cautious |
17:00-18:00 | Invited Talk: Peter Stone (UT Austin) Practical Reinforcement Learning: Lessons from 30 years of Research |
~19:00 | Social Gathering The Blind Beggar Pub (Google Maps) |
Tuesday May 30
08:30-10:00 | Session IV - Chair: Connor Yates |
08:30-09:30 | Invited Talk: Chris Amato (Northeastern University) Principled and Scalable Multi-Agent Reinforcement Learning |
09:30-09:45 | Philipp Altmann, Thomy Phan, Fabian Ritz, Claudia Linnhoff-Popien and Thomas Gabor DIRECT: Learning from Sparse and Shifting Rewards using Discriminative Reward Co-Training |
09:45-10:00 | Henrik Müller, Lukas Berg and Daniel Kudenko Using Incomplete and Incorrect Plans to Shape Reinforcement Learning in Long-Sequence Sparse-Reward Tasks |
10:00-11:00 | Coffee Break |
11:00-12:30 | Session V - Chair: Roxana Rădulescu |
11:00-11:15 | Guanbao Yu, Umer Siddique and Paul Weng Fair Deep Reinforcement Learning with Generalized Gini Welfare Functions |
11:15-11:30 | Long Talk: Manel Rodriguez-Soto, Roxana Radulescu, Juan Antonio Rodriguez Aguilar, Maite Lopez-Sanchez and Ann Nowe Multi-objective reinforcement learning for guaranteeing alignment with multiple values |
11:30-12:30 | Short Talks, 6 minutes each in order:
|
12:30-14:00 | Lunch Break |
14:00-15:45 | Short Talks & Poster Session - Chair: Gaurav Dixit |
14:00-14:15 | Short Talks, 6 minutes each in order:
|
14:15-15:45 | Poster Session B |
15:45-16:30 | Coffee Break |
16:30-18:00 | Session VI - Chair: Diederik M. Roijer |
16:30-16:45 | Long Talk: Alain Andres, Lukas Schäfer, Esther Villar-Rodriguez, Stefano Albrecht and Javier Del Ser Using Offline Data to Speed-up Reinforcement Learning in Procedurally Generated Environments |
16:45-17:00 | Long Talk: Adam Callaghan, Karl Mason and Patrick Mannion Evolutionary Strategy guided Reinforcement Learning via MultiBuffer Communication |
17:00-18:00 | Panel Discussion With Invited Speakers |
18:00 | Awards & Closing Remarks |
Poster Session A - Monday May 29 14:15-15:45
All papers presented on day 1, together with:
- Armaan Garg and Shashi Shekhar Jha
Autonomous Flood Area Coverage using Decentralized Multi-UAV System with Directed Explorations - Seán Caulfield Curley, Karl Mason and Patrick Mannion
A Classification Based Approach to Identifying and Mitigating Adversarial Behaviours in Deep Reinforcement Learning Agents - Sebastian Schmid and Andreas Harth
Distributed Fault Detection For Multi-Agent Systems Based On Vertebrate Foraging - Maxime Toquebiau, Nicolas Bredeche, Faïz Ben Amar and Jae Yun Jun Kim
Joint Intrinsic Motivation for Coordinated Exploration in Multi-Agent Reinforcement Learning - Ridhima Bector, Hang Xu, Abhay Aradhya, Chai Quek and Zinovi Rabinovich
Should Importance of an Attack's Future be Determined by its Current Success? - Xue Yang, Enda Howley and Michael Schukat
ADT: Agent-based Dynamic Thresholding for Anomaly Detection - Abilmansur Zhumabekov, Daniel May, Tianyu Zhang, Aakash Krishna, Omid Ardakanian and Matthew Taylor
Ensembling Diverse Policies Improves Generalizability of Reinforcement Learning Algorithms in Continuous Control Tasks - Alexandra Cimpean, Pieter Libin, Youri Coppens, Catholijn Jonker and Ann Nowé
Towards Fairness In Reinforcement Learning - Anna Penzkofer, Simon Schaefer, Florian Strohm, Mihai Bâce, Stefan Leutenegger and Andreas Bulling
Int-HRL: Towards Intention-based Hierarchical Reinforcement Learning - Yuan Xue, Megha Khosla and Daniel Kudenko
Regulating Action Value Estimation in Deep Reinforcement Learning - Yuxuan Li, Qinglin Liu, Nan Lin and Matthew Taylor
Work in Progress: Integrating Human Preference and Human Feedback for Environmentally Adaptable Robotic Learning
Poster Session B - Tuesday May 30 14:15-15:45
All papers presented on day 2, together with:
- Bruno Rodrigues, Matthias Knorr, Ludwig Krippahl and Ricardo Gonçalves
Towards Explaining Actions of Learning Agents - Ridhima Bector, Hang Xu, Abhay Aradhya, Chai Quek and Zinovi Rabinovich
Poisoning the Well: Can We Simultaneously Attack a Group of Learning Agents? - Junlin Lu, Patrick Mannion and Karl Mason
Inferring Preferences from Demonstrations in Multi-objective Reinforcement Learning: A Dynamic Weight-based Approach - Louis Bagot, Lynn D'Eer, Steven Latre, Tom De Schepper and Kevin Mets
GPI-Tree Search: Algorithms for Decision-time Planning with the General Policy Improvement Theorem - Archana Vadakattu, Michelle Blom and Adrian Pearce
Strategy Extraction in Single-agent Games - Danila Valko and Daniel Kudenko
Increasing Energy Efficiency of Bitcoin Infrastructure with Reinforcement Learning and One-shot Path Planning for the Lightning Network - Jacobus Smit and Fernando P. Santos
Learning Fair Cooperation in Systems of Indirect Reciprocity - Md. Saiful Islam, Srijita Das, Sai Krishna Gottipati, William Duguay, Cloderic Mars, Jalal Arabneydi, Antoine Fagette, Matthew Guzdial and Matthew E. Taylor
WIP: Human-AI interactions in real-world complex environments using a comprehensive reinforcement learning framework - Ward Gauderis, Fabian Denoodt, Bram Silue, Pierre Vanvolsem and Andries Rosseau
Efficient Bayesian Ultra-Q Learning for Multi-Agent Games - Richard Willis and Michael Luck
Resolving social dilemmas through reward transfer commitments - Udari Madhushani, Kevin McKee, John Agapiou, Joel Leibo, Richard Everett, Thomas Anthony, Edward Hughes, Karl Tuyls and Edgar Duenez-Guzman
Heterogeneous Social Value Orientation Improves Meaningful Diversity in Various Incentive Structures
Accepted Papers
Paper # | Authors | Title |
---|---|---|
2 | Lin Shi and Bei Peng | Curriculum Learning for Relative Overgeneralization |
3 | Felipe Leno Da Silva, Jiachen Yang, Mikel Landajuela, Andre Goncalves, Alexander Ladd, Daniel Faissol and Brenden Petersen | Toward Multi-Fidelity Reinforcement Learning for Symbolic Optimization |
7 | Armaan Garg and Shashi Shekhar Jha | Autonomous Flood Area Coverage using Decentralized Multi-UAV System with Directed Explorations |
8 | Montaser Mohammedalamen, Dustin Morrill, Alexander Sieusahai, Yash Satsangi and Michael Bowling | Learning to Be Cautious |
9 | Seán Caulfield Curley, Karl Mason and Patrick Mannion | A Classification Based Approach to Identifying and Mitigating Adversarial Behaviours in Deep Reinforcement Learning Agents |
10 | Malek Mechergui and Sarath Sreedharan | Goal Alignment: Re-analyzing Value Alignment Problems Using Human-Aware AI |
12 | Qian Shao, Pradeep Varakantham and Shih-Fen Cheng | Cost Constrained Imitation Learning |
13 | Sebastian Schmid and Andreas Harth | Distributed Fault Detection For Multi-Agent Systems Based On Vertebrate Foraging |
14 | Bruno Rodrigues, Matthias Knorr, Ludwig Krippahl and Ricardo Gonçalves | Towards Explaining Actions of Learning Agents |
15 | Manel Rodriguez-Soto, Roxana Radulescu, Juan Antonio Rodriguez Aguilar, Maite Lopez-Sanchez and Ann Nowe | Multi-objective reinforcement learning for guaranteeing alignment with multiple values |
16 | Maxime Toquebiau, Nicolas Bredeche, Faïz Ben Amar and Jae Yun Jun Kim | Joint Intrinsic Motivation for Coordinated Exploration in Multi-Agent Reinforcement Learning |
17 | Minae Kwon, John Agapiou, Edgar Duéñez-Guzmán, Romuald Elie, Georgios Piliouras, Kalesha Bullard and Ian Gemp | Aligning Local Multiagent Incentives with Global Objectives |
18 | Ridhima Bector, Hang Xu, Abhay Aradhya, Chai Quek and Zinovi Rabinovich | Should Importance of an Attack's Future be Determined by its Current Success? |
19 | Ridhima Bector, Hang Xu, Abhay Aradhya, Chai Quek and Zinovi Rabinovich | Poisoning the Well: Can We Simultaneously Attack a Group of Learning Agents? |
20 | Junlin Lu, Patrick Mannion and Karl Mason | Inferring Preferences from Demonstrations in Multi-objective Reinforcement Learning: A Dynamic Weight-based Approach |
21 | Simon Vanneste, Astrid Vanneste, Tom De Schepper, Siegfried Mercelis, Peter Hellinckx and Kevin Mets | Distributed Critics using Counterfactual Value Decomposition in Multi-Agent Reinforcement Learning |
22 | Philipp Altmann, Thomy Phan, Fabian Ritz, Claudia Linnhoff-Popien and Thomas Gabor | DIRECT: Learning from Sparse and Shifting Rewards using Discriminative Reward Co-Training |
23 | Callum Rhys Tilbury, Filippos Christianos and Stefano V. Albrecht | Revisiting the Gumbel-Softmax in MADDPG |
24 | Louis Bagot, Lynn D'Eer, Steven Latre, Tom De Schepper and Kevin Mets | GPI-Tree Search: Algorithms for Decision-time Planning with the General Policy Improvement Theorem |
25 | Seongmin Kim, Woojun Kim, Jeewon Jeon, Youngchul Sung and Seungyul Han | Off-Policy Multi-Agent Policy Optimization with Multi-Step Counterfactual Advantage Estimation |
26 | Xue Yang, Enda Howley and Michael Schukat | ADT: Agent-based Dynamic Thresholding for Anomaly Detection |
27 | Nicole Orzan, Erman Acar, Davide Grossi and Roxana Rădulescu | Emergent Cooperation and Deception in Public Good Games |
28 | Henrik Müller, Lukas Berg and Daniel Kudenko | Using Incomplete and Incorrect Plans to Shape Reinforcement Learning in Long-Sequence Sparse-Reward Tasks |
29 | Matthew E. Taylor | Reinforcement Learning Requires Human-in-the-Loop Framing and Approaches |
30 | Adam Callaghan, Karl Mason and Patrick Mannion | Evolutionary Strategy guided Reinforcement Learning via MultiBuffer Communication |
31 | Abilmansur Zhumabekov, Daniel May, Tianyu Zhang, Aakash Krishna, Omid Ardakanian and Matthew Taylor | Ensembling Diverse Policies Improves Generalizability of Reinforcement Learning Algorithms in Continuous Control Tasks |
32 | Isuri Perera, Frits de Nijs and Julian Garcia | Learning to cooperate against ensembles of diverse opponents |
33 | Rory Lipkis and Adrian Agogino | Discovery and Analysis of Rare High-Impact Failure Modes Using Adversarial RL-Informed Sampling |
34 | Guanbao Yu, Umer Siddique and Paul Weng | Fair Deep Reinforcement Learning with Generalized Gini Welfare Functions |
35 | Archana Vadakattu, Michelle Blom and Adrian Pearce | Strategy Extraction in Single-agent Games |
37 | Lukas Schäfer, Oliver Slumbers, Stephen McAleer, Yali Du, Stefano Albrecht and David Mguni | Ensemble Value Functions for Efficient Exploration in Multi-Agent Reinforcement Learning |
38 | Robert Loftin, Mustafa Mert Çelikok, Herke Van Hoof, Samuel Kaski and Frans Oliehoek | Uncoupled Learning of Differential Stackelberg Equilibria with Commitments |
39 | Alexandra Cimpean, Pieter Libin, Youri Coppens, Catholijn Jonker and Ann Nowé | Towards Fairness In Reinforcement Learning |
40 | Danila Valko and Daniel Kudenko | Increasing Energy Efficiency of Bitcoin Infrastructure with Reinforcement Learning and One-shot Path Planning for the Lightning Network |
41 | Nicola Mc Donnell, Enda Howley and Jim Duggan | QD(λ) Learning: Towards Multi-agent Reinforcement Learning for Learning Communication Protocols |
42 | Mathieu Reymond, Florent Delgrange, Guillermo A. Pérez and Ann Nowé | WAE-PCN: Wasserstein-autoencoded Pareto Conditioned Networks |
44 | Changxi Zhu, Mehdi Dastani and Shihan Wang | Continuous Communication with Factorized Policy Gradients in Multi-agent Deep Reinforcement Learning |
45 | Jannis Weil, Johannes Czech, Tobias Meuser and Kristian Kersting | Know your Enemy: Investigating Monte-Carlo Tree Search with Opponent Models in Pommerman |
46 | Sotirios Nikoloutsopoulos, Iordanis Koutsopoulos and Michalis Titsias | Personalized Federated Learning with Exact Distributed Stochastic Gradient Descent Updates |
47 | Alain Andres, Lukas Schäfer, Esther Villar-Rodriguez, Stefano Albrecht and Javier Del Ser | Using Offline Data to Speed-up Reinforcement Learning in Procedurally Generated Environments |
48 | Yash Satsangi and Paniz Behboudian | Bandit-Based Policy Invariant Explicit Shaping |
50 | Johan Källström and Fredrik Heintz | Model-Based Multi-Objective Reinforcement Learning with Dynamic Utility Functions |
51 | Dimitris Michailidis, Willem Röpke, Sennay Ghebreab, Diederik M. Roijers and Fernando P. Santos | Fairness in Transport Network Design - A Multi-Objective Reinforcement Learning Approach |
52 | Raphael Avalos, Florent Delgrange, Ann Nowe, Guillermo A. Pérez and Diederik M. Roijers | The Wasserstein Believer: Learning Belief Updates for Partially Observable Environments through Reliable Latent Space Models |
53 | Jacobus Smit and Fernando P. Santos | Learning Fair Cooperation in Systems of Indirect Reciprocity |
54 | Kartik Bharadwaj, Chandrashekar Lakshminarayanan and Balaraman Ravindran | Continuous Tactical Optimism and Pessimism |
55 | Prashank Kadam, Ruiyang Xu and Karl Lieberherr | Accelerating Neural MCTS Algorithms using Neural Sub-Net Structures |
56 | Md. Saiful Islam, Srijita Das, Sai Krishna Gottipati, William Duguay, Cloderic Mars, Jalal Arabneydi, Antoine Fagette, Matthew Guzdial and Matthew E. Taylor | WIP: Human-AI interactions in real-world complex environments using a comprehensive reinforcement learning framework |
57 | Ward Gauderis, Fabian Denoodt, Bram Silue, Pierre Vanvolsem and Andries Rosseau | Efficient Bayesian Ultra-Q Learning for Multi-Agent Games |
59 | Anna Penzkofer, Simon Schaefer, Florian Strohm, Mihai Bâce, Stefan Leutenegger and Andreas Bulling | Int-HRL: Towards Intention-based Hierarchical Reinforcement Learning |
60 | Alex Goodall and Francesco Belardinelli | Approximate Shielding of Atari Agents for Safe Exploration |
62 | Yuan Xue, Megha Khosla and Daniel Kudenko | Regulating Action Value Estimation in Deep Reinforcement Learning |
63 | David Radke and Kyle Tilbury | Learning to Learn Group Alignment: A Self-Tuning Credo Framework with Multiagent Teams |
64 | Yuxuan Li, Qinglin Liu, Nan Lin and Matthew Taylor | Work in Progress: Integrating Human Preference and Human Feedback for Environmentally Adaptable Robotic Learning |
65 | Richard Willis and Michael Luck | Resolving social dilemmas through reward transfer commitments |
67 | Udari Madhushani, Kevin McKee, John Agapiou, Joel Leibo, Richard Everett, Thomas Anthony, Edward Hughes, Karl Tuyls and Edgar Duenez-Guzman | Heterogeneous Social Value Orientation Improves Meaningful Diversity in Various Incentive Structures |
69 | Luis Thomasini, Lucas Alegre, Gabriel De O. Ramos and Ana L. C. Bazzan | RouteChoiceGym: a Route Choice Library for Multiagent Reinforcement Learning |
Invited Talks
Shimon Whiteson
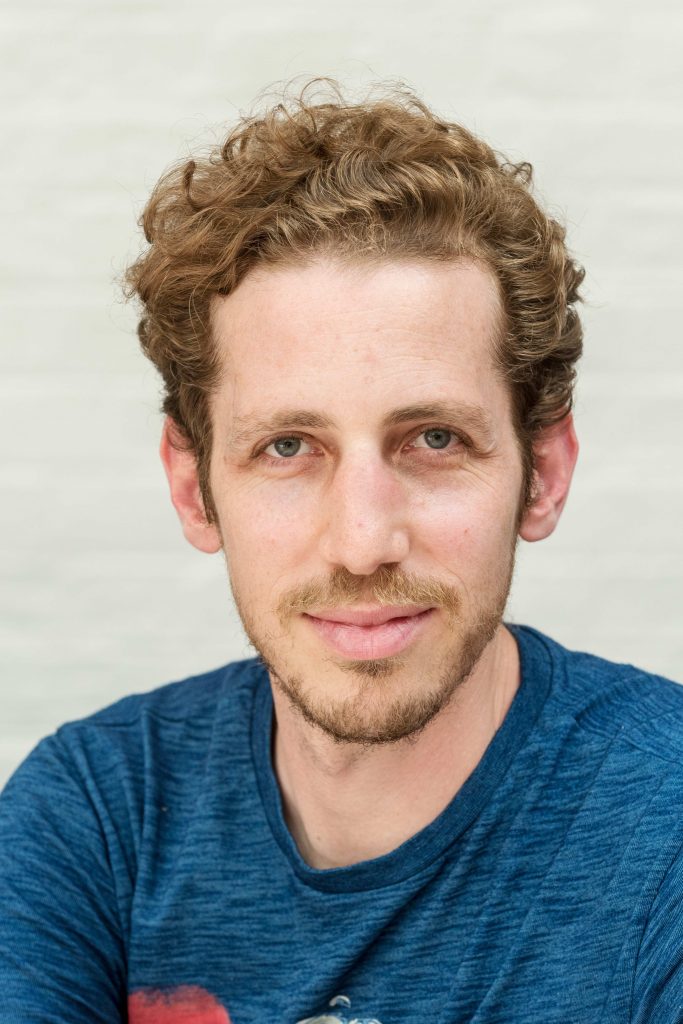
Affiliation: University of Oxford, Waymo UK
Website: http://whirl.cs.ox.ac.uk/index.html
Title: Title: Efficient & Realistic Simulation for Autonomous Driving
Abstract: In this talk, I will discuss some of the key challenges in performing efficient and realistic simulation for autonomous driving, with a particular focus on how to train simulated agents that model the human road users, such as cars, cyclists, and pedestrians who share the road with autonomous vehicles. I will discuss the need for distributionally realistic agents and present two methods for training hierarchical agents to this end. Finally, I will discuss how the resulting simulator can be used to efficiently train a planning agent to control the autonomous vehicle itself.
Bio: Shimon Whiteson is a Professor of Computer Science at the University of Oxford and the Head of Research at Waymo UK. His research focuses on deep reinforcement learning and learning from demonstration, with applications in robotics and video games. He completed his doctorate at the University of Texas at Austin in 2007. He spent eight years as an Assistant and then an Associate Professor at the University of Amsterdam before joining Oxford as an Associate Professor in 2015. He was awarded a Starting Grant from the European Research Council in 2014, a Google Faculty Research Award in 2017, and a JPMorgan Faculty Award in 2019.
Peter Stone
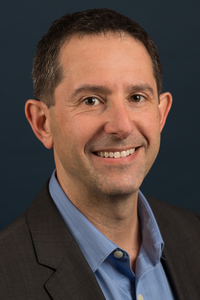
Affiliation: The University of Texas at Austin
Website: https://www.cs.utexas.edu/~pstone/
Title: Practical Reinforcement Learning: Lessons from 30 years of Research
Abstract: The field of reinforcement learning (RL) has a long history of theoretical results that indicate when RL algorithms *should* work. Throughout this history, there has been a complementary thread of research that tests the theoretical assumptions by seeking to determine when (and how) RL algorithms *do* work in practice. Drawing on 30 years of research results, mostly from the Learning Agents Research Group at UT Austin, this talk will summarize lessons learned about practical RL into four high-level topics: 1) Representation - choosing the algorithm for the problem's representation and adapating the representation to fit the algorithm; 2) Interaction - with other agents and with human trainers; 3) Synthesis - of different algorithms for the same problem and of different concepts in the same algorithm; and 4) Mortality - dealing with the constraint that in most practical settings, opportunities for learning experience are limited. The talk will conclude with a brief introduction to one of the largest ever commercial deployments of an RL agent, Gran Turismo Sophy, which in 2021 won a head-to-head competition against four of the world's best drivers in the Gran Turismo high speed racing game.
Bio: Dr. Peter Stone holds the Truchard Foundation Chair in Computer Science at the University of Texas at Austin. He is Associate Chair of the Computer Science Department, as well as Director of Texas Robotics. In 2013 he was awarded the University of Texas System Regents' Outstanding Teaching Award and in 2014 he was inducted into the UT Austin Academy of Distinguished Teachers, earning him the title of University Distinguished Teaching Professor. Professor Stone's research interests in Artificial Intelligence include machine learning (especially reinforcement learning), multiagent systems, and robotics. Professor Stone received his Ph.D in Computer Science in 1998 from Carnegie Mellon University. From 1999 to 2002 he was a Senior Technical Staff Member in the Artificial Intelligence Principles Research Department at AT&T Labs - Research. He is an Alfred P. Sloan Research Fellow, Guggenheim Fellow, AAAI Fellow, IEEE Fellow, AAAS Fellow, ACM Fellow, Fulbright Scholar, and 2004 ONR Young Investigator. In 2007 he received the prestigious IJCAI Computers and Thought Award, given biannually to the top AI researcher under the age of 35, and in 2016 he was awarded the ACM/SIGAI Autonomous Agents Research Award. Professor Stone co-founded Cogitai, Inc., a startup company focused on continual learning, in 2015, and currently serves as Executive Director of Sony AI America.
Christopher Amato
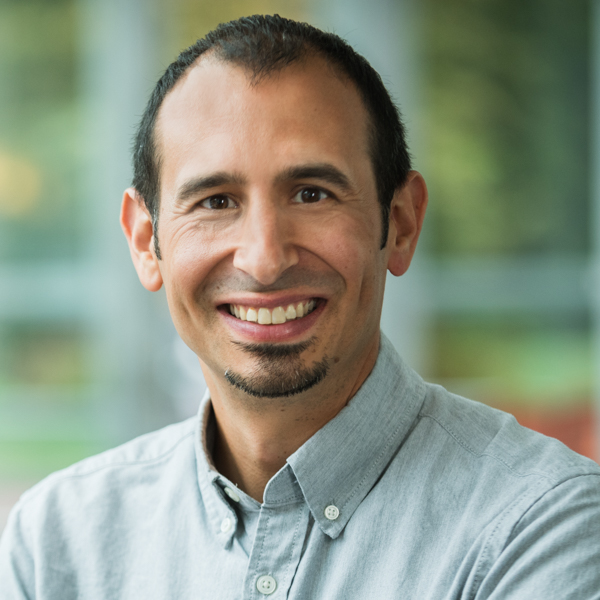
Affiliation:Northeastern University
Talk Title: Principled and Scalable Multi-Agent Reinforcement Learning
Abstract: The decreasing cost and increasing sophistication of hardware has created new opportunities for applications where teams of agents (e.g., robots, autonomous vehicles, AI software) can be deployed to solve complex problems. However, if such systems are to become widely deployable, we must develop the control and coordination methods that allow the agents to perform well in large real-world domains with significant uncertainty and communication limitations. I’ll first talk about some of the fundamental challenges and misunderstandings of multi-agent reinforcement learning along with promising future directions. In particular, I’ll discuss how 1) centralized critics are not strictly better than decentralized critics (and can be worse), and 2) state-based critics are unsound and work well only on a subclass of problems. I’ll also talk about some of our work on scalable reinforcement learning methods for asynchronous multi-agent systems.
Bio: Christopher Amato is an Associate Professor at Northeastern University where he leads the Lab for Learning and Planning in Robotics. He has published over 60 papers in leading artificial intelligence, machine learning and robotics conferences (including winning a best paper prize at AAMAS-14 and being nominated for the best paper at RSS-15, AAAI-19, AAMAS-21 and MRS-21). He has also won several awards such as Amazon Research Awards and an NSF CAREER Award. His research focuses on reinforcement learning in partially observable and multi-agent/multi-robot systems.
Awards
Best Paper Award
We are pleased to announce the best paper of ALA 2023, sponsored by Neural Computing & Applications, is Fair Deep Reinforcement Learning with Generalized Gini Welfare Functions, by Guanbao Yu, Umer Siddique and Paul Weng!
Programe Committee
- Adrian Agogino, University of California Santa Cruz, USA
- Lucas Alegre, UFRGS, BRA
- Muhammad Arrasy-Rahman, The University of Texas at Austin, USA
- Raphael Avalos, Vrije Universiteit Brussel, BEL
- Angel Ayala, Universidade de Pernambuco, BRA
- Wolfram Barfuss, Tuebingen AI Center, University of Tuebingen, GER
- Rodrigo Bonini, Federal University of ABC (UFABC), BRA
- Roland Bouffanais, University of Ottawa, CAN
- Adam Callaghan, University of Galway, IRL
- Mustafa Mert Çelikok, Aalto University FIN
- Raphael Cobe, Sao Paulo State University, BRA
- Francisco Cruz, Deakin, AUS
- Jiaxun Cui, The University of Texas at Austin, USA
- Felipe leno Da silva, LLNL, USA
- Gaurav Dixit, Oregon State University, USA
- Florian Felten, SnT, University of Luxembourg, LUX
- Elias Fernández Domingos, MLG, Université Libre de Bruxelles; AI-lab, Vrije Universiteit Brussels, BEL
- Julian Garcia, Monash University, AUS
- Ruben Glatt, Lawrence Livermore National Laboratory, USA
- Brent Harrison, University of Kentucky, USA
- Conor F Hayes, NUI Galway, IRL
- Thommen Karimpanal George, Deakin University, AUS
- Sammie Katt, Northeastern University, USA
- Matt Knudson, NASA, USA
- Johan Källström, Linköping University, SWE
- Mikel Landajuela Larma, Lawrence Livermore National Laboratory, USA
- Junlin Lu, University of Galway, IRL
- Udari Madhushani, Princeton University, USA
- Patrick Mannion, National University of Ireland Galway, IRL
- Karl Mason, University of Galway, IRL
- Nicolás Navarro-Guerrero, Deutsches Forschungszentrum für Künstliche Intelligenz (DFKI), GER
- Frans Oliehoek, Delft University of Technology, NLD
- Bei Peng, University of Liverpool, GBR
- Roxana Radulescu, Vrije Universiteit Brussel, BEL
- Gabriel De O. Ramos, Universidade do Vale do Rio dos Sinos, BRA
- Mathieu Reymond, Vrije Universiteit Brussel, BEL
- Diederik M. Roijers, Vrije Universiteit Brussel & HU University of Applied Sciences Utrecht, BEL
- Willem Röpke, Vrije Universiteit Brussel, BEL
- Fernando Santos, University of Amsterdam, NLD
- Miguel Solis, Universidad Andrés Bello, CHL
- Denis Steckelmacher, Vrije Universiteit Brussel, BEL
- Paolo Turrini, University of Warwick, GBR
- Peter Vamplew, Federation University Australia, AUS
- Miguel Vasco, INESC-ID, PRT
- Vítor V. Vasconcelos, University of Amsterdam, NLD
- Caroline Wang, U Texas, USA
- Connor Yates, Oregon State University, USA
- Junpei Zhong, The Hong Kong Polytechnic University, HKG
- Changxi Zhu, Utrecht University, NLD
Organization
This year's workshop is organised by:- Francisco Cruz (UNSW Sydney, AUS)
- Conor F. Hayes (Lawrence Livermore National Laboratory, US)
- Caroline Wang (University of Texas at Austin, US)
- Connor Yates (Oregon State University, US)
- Enda Howley (University of Galway, IE)
- Daniel Kudenko (University of York, UK)
- Patrick Mannion (University of Galway, IE)
- Ann Nowé (Vrije Universiteit Brussel, BE)
- Sandip Sen (University of Tulsa, US)
- Peter Stone (University of Texas at Austin, US)
- Matthew Taylor (University of Alberta, CA)
- Kagan Tumer (Oregon State University, US)
- Karl Tuyls (University of Liverpool, UK)